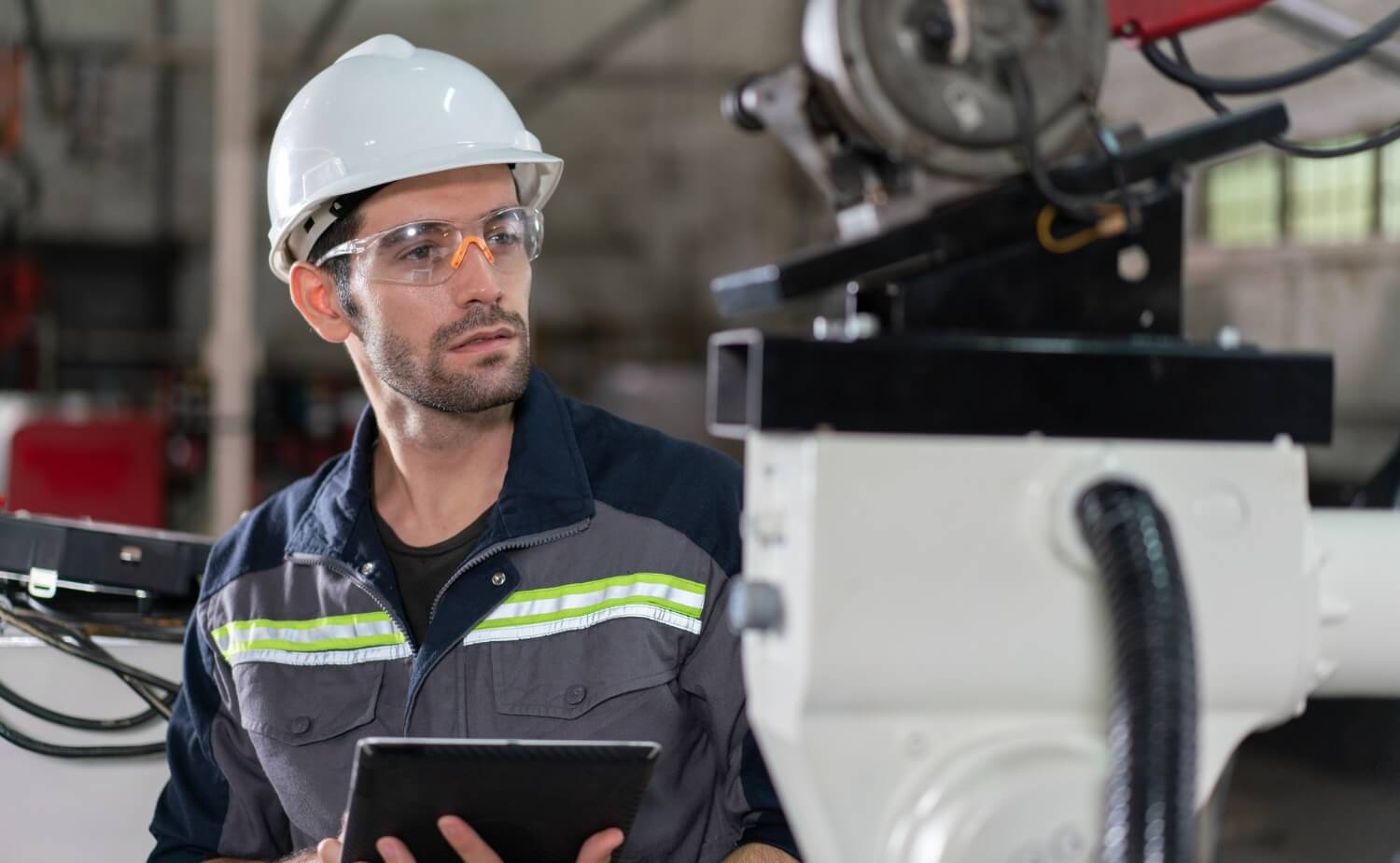
What we see. What we expect. What we implement.
Artificial intelligence (AI) is changing our lives. In recent years in particular, AI has made its presence felt in many people’s everyday lives. Unnoticed, AI has been active in many places for a long time. But what does AI actually look like in industrial production? The use of AI promises great potential for optimization and even completely new approaches. We have taken a look at the status quo from our perspective as a manufacturer and supplier of industrial software.
Where we encounter AI in industry
We have been encountering the use of artificial intelligence in production and industry for many years. Most applications are in specific fields where AI has already proven its worth. Examples include image recognition and predictive maintenance.
Machine vision
Image recognition, also known as machine vision, recognizes objects and thus helps to make decisions in automated processes. It can be used to make quality decisions or to control machine operation.
Machine vision has been in use for many years and has already reached a high level of development. In the background, modern machine vision systems use artificial intelligence methods and techniques such as machine learning or deep learning and artificial neural networks.
Predictive Maintenance
Predictive maintenance combines various strategies to enable proactive maintenance based on findings from process data. In addition to many other data analysis technologies, it may use AI technologies that detect irregularities in data (anomaly detection) or uncover deviations from familiar/learned process sequences (condition monitoring). Predictive maintenance also employs machine learning algorithms running in the background.
What we expect from the use of AI in industry
The topic of AI is present in the minds of decision-makers. The awareness that the use of AI is unavoidable in the medium term is becoming more and more prevalent.
Challenges during implementation
The use of AI in industry is still cautious. In addition to identifying a specific use case, the introduction requires a great deal of expert knowledge and a reliable data basis. Input from different departments is required for comprehensive implementation. In turn, the amortization of investments is not always predictable if it is unclear whether the problem can be solved using AI.
Machine Learning on the rise
The areas of machine vision and predictive maintenance mentioned above show very well how sophisticated AI systems can reliably control and improve processes in individual areas using machine learning. We are already seeing AI systems being introduced in highly specialized departments to solve specific tasks:
- Defect detection in glass production
- Quality assurance based on process data in paper production
- Machine failure prediction through machine data analysis
- Live recommendations to operators for optimized machine parameters
We expect these systems to perform their highly specialized tasks as islands. They will be managed by experts and will eventually be integrated into the industrial production system landscape via standard interfaces. This will then take place as usual using the Industry 4.0 approach, i.e. information for their operation will be transferred from the process to these systems in real time and, in return, the decisions and findings will be retrieved from the system and written back to the production systems.
Domain-specific expert systems
As a result, AI systems will be able to take on more and more specialized tasks. As very specific domain knowledge is required for the individual tasks, we expect to see a very heterogeneous system landscape.
The domain knowledge relates to machine-specific knowledge, product-specific knowledge and also process-specific knowledge. In these use cases, the AI systems must largely be trained with existing and accumulating data in order to meet the specific requirements.
New avenues for AI in production
A new field of AI in production will be large language models. Large language models (LLMs) have become very popular in recent years. In contrast to the specialized machine learning solutions, the existing language models are very well known. Many people are now familiar with the benefits and impressive functions of ChatGPT and the like.
We expect that these systems will also find their place in industrial production and production management. The first application will be assistance systems that help users to evaluate large volumes of production data efficiently. However, language models also have the potential to support production control by helping to generate data thanks to their powerful capabilities. They are able to assist production planning, simplify documentation and realize a new form of operator guidance.
The use of LLMs in production is therefore only just beginning. Unlike machine learning applications, LLMs have already been trained and are available as a ready-made model. This means they can be implemented very quickly.
How manubes is bringing AI to industrial production
With manubes, we have brought artificial intelligence into production via the implementation of language models. The result is our manubes Chat Assistant.
Through the connection to (local) production systems, manubes is continuously supplied with highly relevant production data, which can be written into manubes’ own data models. This data serves as the foundation of our AI-based assistant.
Asking questions about production data
A major strength of generative AI is its ability to convert human questions into technical questions. manubes can answer these technical questions using its production data. The manubes Chat Assistant then uses generative AI to convert the data into answers that can be analyzed by humans and, if requested, even into graphics.
This means that the impressive ability of language models to understand human language well is used directly to answer questions about production. A capability that was previously not available in this form, flexibility and speed. Existing reporting or BI tools always require a certain amount of prior knowledge on the part of the operator and active use of the tools.
AI as an assistant, helper, advisor and soon as an operator
The ability to answer spontaneous questions about production with manubes is already a reality. This is a very powerful functionality. In the future, the plan is to also generate data with this function. This will be the next step in the use of language models in manubes and therefore another very exciting use of AI in production. AI, and therefore manubes, will become active players in production. They will become virtual operators.
Recent Comments